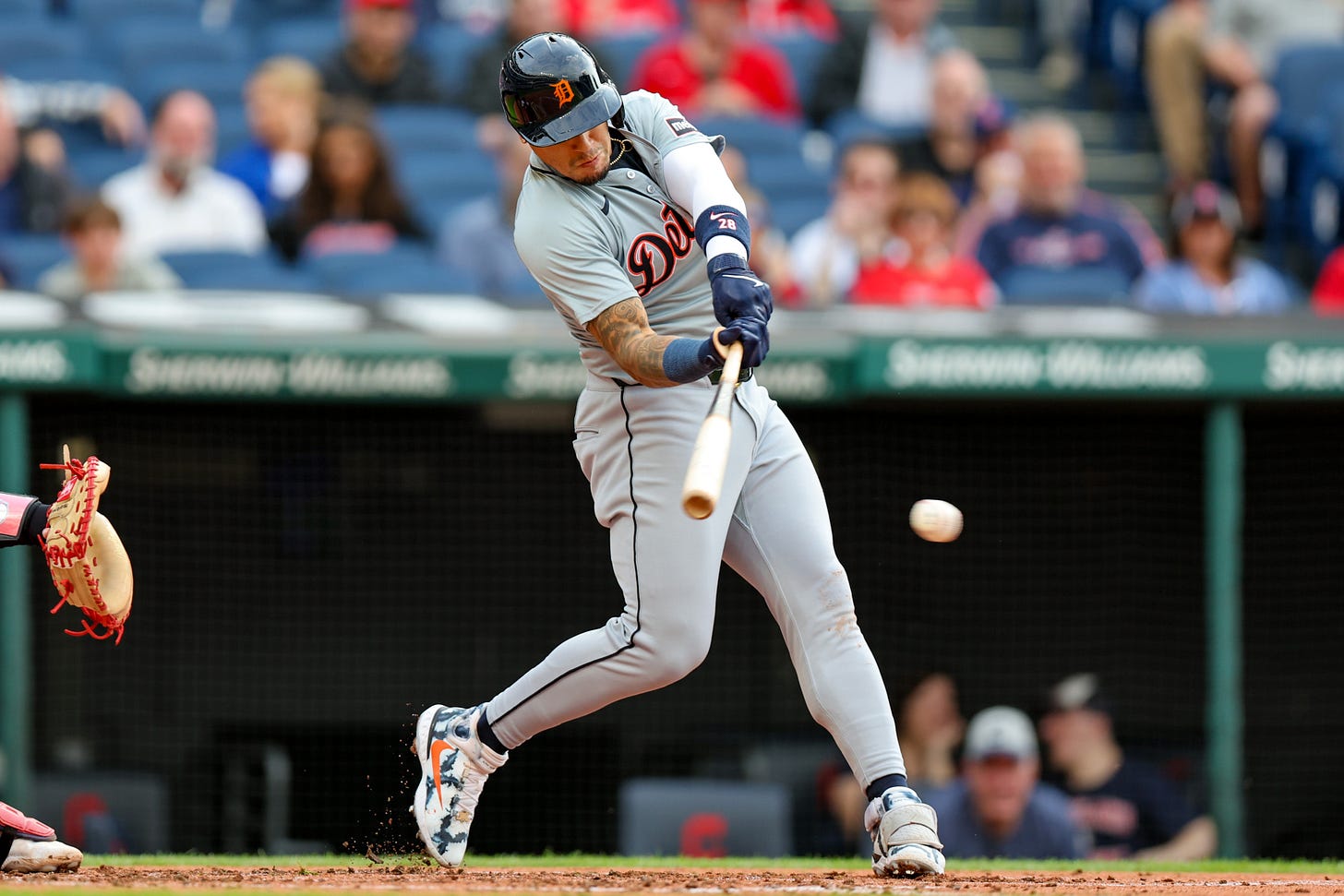
The baseball corner of the internet was abuzz a few days ago after MLB released a new class of Statcast metrics over on Baseball Savant: Bat tracking data.
The new metrics, which can be found in leaderboards here and also among Baseball Savant’s percentile grades, measure the speed and path of the bat as a hitter swings using Hawk-Eye cameras — as well as how much the sweet spot of the bat was squared up with the ball (based on how much potential power translated to actual exit velocity). It’s all very cool, and yet another application of the type of tracking technology we’ve seen reshape sports over the past decade or so.
But this new data got me wondering: How much does bat speed really matter?
Here’s a plot of this season’s percentile grades in bat speed versus expected wOBA, a luck-neutral measure of hitting performance based (mostly) on exit velocity and launch angle:
As you might suspect from looking at that crazy scatter, the correlation between bat speed and xWOBA percentiles is 0.274, meaning that swinging hard explains only about 8% of batting performance even if we take out luck and defense as confounding factors.
That makes sense intuitively when we simply look at the leaderboard for bat speed, too. Some guys swing hard and it leads to good results — Kyle Schwarber, Christopher Morel, Ronald Acuña Jr., Aaron Judge, Jo Adell and Juan Soto are all among the top 10 in swing speed and the top third of hitters in xWOBA. But others, such as Oneil Cruz, Giancarlo Stanton (who is the god of this new bat-speed metric), Matt Chapman, Jorge Soler and, oddly, Julio Rodríguez swing very hard to get mediocre results at best.
Then there are guys like Javier Báez and Elehuris Montero, who have some of the best bat speeds in the game — and some of the worst outcomes.
So clearly, there’s a lot more to hitting than swinging the bat hard. (And, in fairness, the Statcast folks never claimed otherwise! This is just one more cool feature to track about a player’s game.) For instance, if all you knew about a player was his average bat speed and how frequently he hit the ball with the optimal launch angle, having the correct launch angle would be 2.3 times as important when predicting a player’s xWOBA.
What maybe stood out as most surprising to me in this data-dive, though, is just how little correlation there is between bat speed and exit velocity:
The correlation coefficient here is certainly higher, at 0.545, than it was for xWOBA. (This makes sense, because xWOBA accounts for many things beyond just how hard the ball is swung at and/or hit.) But it still strikes me as surprising that bat speed only explains roughly 30% of the variation in exit velocity, since I think most fans would assume that swinging hard automatically leads to hard-hit balls when contact is made.
And yet, plenty of players have strange disconnects between their bat speed and average EV: Mookie Betts, for example, is in the 17th percentile of bat speed but the 75th percentile of EV (and the 95th percentile of xWOBA!). Out of 218 qualified players, 55 are out velo-ing their bat speed by at least 20 percentile points, and 29 are doing it by at least 30 points. At the other end of things, we have players like Corbin Carroll, who has 83rd-percentile bat speed but 15th percentile exit velocity (and 41st-percentile xWOBA). In total, 43 players have an EV percentile at least 20 points below their bat speed percentile, and 28 are running at least 30 points lower.
The correlation is higher (0.738, or a 54.5% R-squared) for bat speed versus max exit velo, so it does seem like faster swings indicate more hard-hitting potential. (That may end up being the most useful application here: a way to find players with room to improve via coaching/technique.) But overall, better bat speed doesn’t necessarily mean better batting performance — nor does it even guarantee you’ll consistently hit the ball significantly harder, surprisingly enough.
Filed under: Baseball
In 1976, while sitting in our family car and talking incessantly about how otherworldly Doctor J. was, my father turned to me and said to me "son, we all stand on the shoulders of giants of who came before us. Without Elgin Baylor, there would be no Doctor J." I had never heard of Elgin Baylor and left the car unconvinced.
Fast forward to 1985 when as I am strolling through Von's Books in West Lafayette, Indiana a book on the front table catches my eye. It was entitled "Sports Science - Physical Laws and Optimum Performance" by Peter J. Brancazio, a physics professor at Brooklyn College. The book combined my two passions - sports and science. I quickly grabbed a copy and read it cover to cover. I was much smarter when I finished.
What I didn't know then was that "Sports Science" would become a seminal work in the field of sports analytics. While other books soon tried to similarly apply physics to sports, Brancazio's initial work was widely hailed as the best among physicists for its clarity and accuracy in applying the laws of physics. Brancazio was an early sports myth buster and also the creator of new terminology at the time that will be familiar to this audience including "launching angle" and "spin rate."
As I read this piece and others about the excitement and new insights from this data, I immediately recalled how Brancazio had predicted these very same outcomes - 40 years ago. He didn't need fancy and expensive data collection tools to reach the right answers. All he required was his powerful and curious intellect along with the humble, unfailing laws of physics to which the universe had long surrendered...a universe that includes baseball.
Brancazio passed away in April of 2020 - a very early victim of COVID-19. He left behind an enormous legacy for others to be inspired by and to follow. Today's sprawling sports analytic culture owes a large debt to Peter Brancazio and his unassuming little sports book.
In reading the piece, I was struck by two things - how right my father was about those shoulders and how pleased Peter Brancazio would be today to see how his work turned out after all these years.
I think it’ll be interesting to see how predictive it is of future performance - eg Carroll is struggling right now but with his bat speed and short swing length will he start to square up more and then see the outcomes jump (we just have a limited sample right now)